Adventures in machine learning for healthcare
Epic Systems employs artificial intelligence for growing use in medical field.
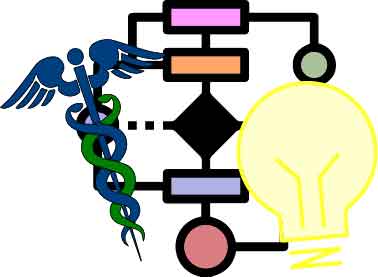
Elizabeth Kaiser graphic
Owen Sizemore, a lead data scientist at Epic, gave a presentation on machine learning in healthcare. Sizemore was an assistant professor of mathematics at University of Wisconsin–Madison before joining the Epic team. He has a bachelor’s degree in Mathematics from University of California-Berkeley and a PhD in Mathematics from University of California-Los Angeles.
Sizemore introduced Epic as an electronic health record company. He explained electronic health records as what doctors use to keep track of their patients’ private data. There has been an increasingly massive digitalization of healthcare data as technology has advanced and become more usable. Therefore, the data that is available keeps growing, too.
“Machine learning is using data that you gather, along with using computer science, math and statistics to teach a computer to do human-like things without explicitly programming the computer to do those things,” Sizemore said.
A survey taken by tech CEOs evaluating the challenges of artificial intelligence found one common concern: these CEOs worry that it will be hard to integrate machine learning into their existing processes. Therefore, Epic has focused on this concern, trying to streamline machine learning to make it more accessible and easier to understand.
Sizemore shared that two of Epic’s more specific focuses are on library and platform. They want to create a library of embedded machine learning models and a platform for running models in real-time.
Sizemore also shared that Epic’s vision for healthcare is getting algorithms on patients as soon as they are admitted to the Emergency Room. These algorithms can be a tool to assist doctors in figuring out what’s wrong. Sizemore presented Lee Health in Fort Myers, Florida, as an example. An Epic predictive model helped Lee Health clinicians identify patterns that indicate adult patients in the early stages of sepsis. This predictive model has helped them reduce mortality by 20% since 2015 by identifying and treating patients in the early stages of sepsis sooner. Research shows that with each hour patients with sepsis don’t receive treatment, their risk of dying increases by 4%, so early detection is critical.
Epic software and machine learning have also helped in eliminating cardiac arrests. Predictions from the software can draw the doctor’s attention to a patient before the cardiac arrest actually happens, allowing for prevention.
With the help of Epic’s machine learning platform and optional integration with Microsoft Azure, Ochsner Health System calculates patients’ risk of deterioration in real time and sends notifications directly to physicians’ mobile devices so they can intervene sooner. This notification system has led to a 44% decrease in cardiac arrests on their floor.
“It’s really about cultural acceptance; I think that there’s a general lack of understanding, not just in the healthcare field but broadly around what machine learning can do now and what it could do in the future. I think that people are too optimistic about what it can do now, and too pessimistic about what it can do in the future. It’s still very early in the field; I think if we could align everyone to understand what we are, then we could actually move forward more quickly,” Sizemore said.
Sizemore shared that Epic is still trying to bridge the gap between their technology and doctors. He explained that machine learning is simply a tool to help doctors, not software meant to replace them. Algorithms can constantly monitor every person in a given medical center, but machine learning is not better than humans at healing. Rather, machine learning can draw attention to a patient so that the doctor can prevent or tend to the problem.